To guarantee accuracy, reliability, and practical insights, it's crucial to examine the AI and machine-learning (ML), models used by prediction and trading platforms. Models that are not properly designed or overhyped could result in financial losses as well as flawed forecasts. Here are ten of the most useful tips to help you evaluate the AI/ML models of these platforms.
1. Understand the model's purpose and the way to apply it.
The goal must be determined. Make sure the model was designed to be used for long-term investment or for trading on a short-term basis.
Algorithm transparency: Check if the platform discloses the types of algorithms employed (e.g. regression and decision trees, neural networks or reinforcement learning).
Customizability - Determine if you can tailor the model to suit your strategy for trading and your risk tolerance.
2. Review the Model Performance Metrics
Accuracy: Verify the accuracy of the model in predicting the future. However, do not solely rely on this metric as it may be misleading when used with financial markets.
Precision and recall (or accuracy) Find out how well your model is able to differentiate between genuine positives - e.g., accurately predicted price fluctuations as well as false positives.
Risk-adjusted return: Determine whether the model's forecasts will yield profitable trades after adjusting for risk (e.g. Sharpe ratio, Sortino coefficient).
3. Test the model by Backtesting
History of performance: The model is tested using historical data in order to assess its performance in previous market conditions.
Examine the model using information that it hasn't been taught on. This will help prevent overfitting.
Scenario analyses: Compare the performance of your model under different markets (e.g. bull markets, bears markets high volatility).
4. Check for Overfitting
Overfitting signs: Look for overfitted models. These are models that do extremely well on training data and poorly on unobserved data.
Regularization methods: Check whether the platform is using techniques such as L1/L2 regularization or dropout in order to prevent overfitting.
Cross-validation: Ensure that the model is cross-validated in order to evaluate the generalizability of the model.
5. Evaluation Feature Engineering
Relevant features: Ensure that the model is based on meaningful features (e.g. price volumes, technical indicators and volume).
Selection of features: Make sure that the system chooses features that are statistically significant. Also, avoid redundant or irrelevant information.
Updates to features that are dynamic Check to see how the model adapts itself to the latest features or changes in the market.
6. Evaluate Model Explainability
Interpretability: Ensure that the model has clear explanations of its predictions (e.g., SHAP values, the importance of features).
Black-box model: Beware of platforms which employ models that are overly complicated (e.g. deep neural network) without describing methods.
User-friendly Insights: Make sure that the platform provides useful information in a format that traders are able to easily comprehend and use.
7. Assessing Model Adaptability
Market fluctuations: See whether your model is able to adapt to market fluctuations (e.g. new rules, economic shifts, or black-swan events).
Continuous learning: Determine whether the platform continually updates the model to include the latest data. This can boost performance.
Feedback loops: Ensure that the platform is able to incorporate real-world feedback as well as user feedback to improve the design.
8. Be sure to look for Bias in the elections
Data bias: Ensure that the information provided used in the training program are accurate and does not show bias (e.g., a bias toward certain industries or times of time).
Model bias - Check to see if your platform actively monitors the presence of biases within the model predictions.
Fairness: Ensure that the model does not disproportionately favor or disadvantage specific stocks, sectors, or trading styles.
9. Assess Computational Effectiveness
Speed: Check if a model can produce predictions in real time with the least latency.
Scalability: Determine whether the platform can manage several users and massive datasets without performance degradation.
Resource usage: Check if the model is optimized to utilize computational resources efficiently (e.g. the GPU/TPU utilization).
Review Transparency and Accountability
Model documentation: Make sure the platform has comprehensive documentation about the model's design and its training process.
Third-party auditors: Make sure whether the model has been subject to an audit by an independent party or has been validated by an outside party.
Error handling: Determine that the platform has mechanisms to detect and rectify models that have failed or are flawed.
Bonus Tips
Case studies and user reviews User reviews and case studies: Study feedback from users as well as case studies in order to gauge the model's performance in real life.
Trial period: Try the model free of charge to determine how accurate it is as well as how easy it is to utilize.
Support for customers: Make sure that the platform can provide robust customer support to help solve any product or technical issues.
These tips will help you evaluate the AI and machine learning models employed by platforms for prediction of stocks to ensure they are reliable, transparent and compatible with your objectives in trading. Follow the best ai investing tips for more examples including ai investment platform, market ai, chart ai trading assistant, best ai stock, ai stock, ai stock trading app, ai stock market, market ai, best ai for trading, ai for investment and more.
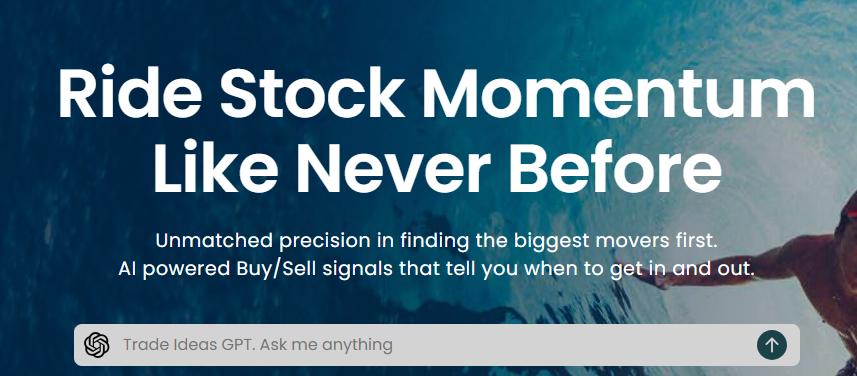
Top 10 Tips For Assessing The Reputation, Reviews And Reviews Of Ai-Powered Stock Trading Platforms
In order to guarantee security, reliability, efficacy and trustworthiness It is essential to check the reviews and reputation of AI-powered platform for prediction and trading stocks. Below are the top 10 tips to evaluate the reputation and reviews.
1. Check Independent Review Platforms
Tips: Search for reviews on trusted platforms like G2, copyright, or Capterra.
The reason is that independent platforms allow users to provide feedback that is unbiased.
2. Review User Testimonials as well as Study Case Studies
Tips: Read testimonials from users and case studies on the platform's site or other third-party sites.
What are the benefits? They provide insight into the real-world performance of a system and the level of satisfaction among users.
3. Review of Expert Opinions Industry Recognition
Tip: Find out whether any experts in the field, analysts, or publications with a reputation have reviewed the platform, or given it a recommendation.
Why: Expert endorsements add credibility to the claims of the platform.
4. Assess Social Media Sentiment
Tip: Monitor social media platforms such as Twitter, LinkedIn or Reddit for comments and sentiments from users.
Social media provides you with the an opportunity to listen to thoughts and opinions that aren't filtering.
5. Verify whether the regulation is compliant
TIP: Make sure that the platform is in compliance with financial laws (e.g., SEC, FINRA) and the laws governing data privacy (e.g. GDPR, e.g.).
Why: Compliance ensures that the platform functions legally and with integrity.
6. Transparency is a key element in performance metrics
Tip: Check if the platform offers transparent performance metrics, like accuracy rates, ROI, and backtesting results.
The reason: Transparency creates trust and helps users evaluate the effectiveness of the platform.
7. Verify the quality of customer support.
Read reviews about the platform to learn about their customer service.
Why is this? A reliable customer support service is crucial for resolving any issues and providing a positive customer experience.
8. Red Flags are a good indicator of a negative review
Tips - Watch out for recurring complaints like low performance, hidden costs, or lack updates.
If you have a pattern of bad feedback, it can indicate that there may be an issue in the system.
9. Evaluate Community and User Engagement
Tips: Ensure that the platform is active and is regularly engaging users (e.g. forums, Discord groups).
The reason is that a strong user base is a sign of satisfaction and support.
10. Check the company's track record
Tips: Research the company's history, leadership team, and past performances in the field of financial technology.
What's the reason? A track record can increase confidence in the platform’s reliability and expertise.
Compare multiple platforms
Compare reviews and the reputations of multiple platforms to identify the most suitable one for your requirements.
By following these guidelines, it is possible to evaluate and review the reputations and reviews of AI-based trading and stock prediction solutions to ensure that you select the most reliable and effective solution. View the recommended more help on chart analysis ai for more info including best stock prediction website, investing with ai, ai stock prediction, ai stock price prediction, chart analysis ai, ai stock predictions, ai investment tools, ai for trading stocks, trading ai tool, free ai tool for stock market india and more.
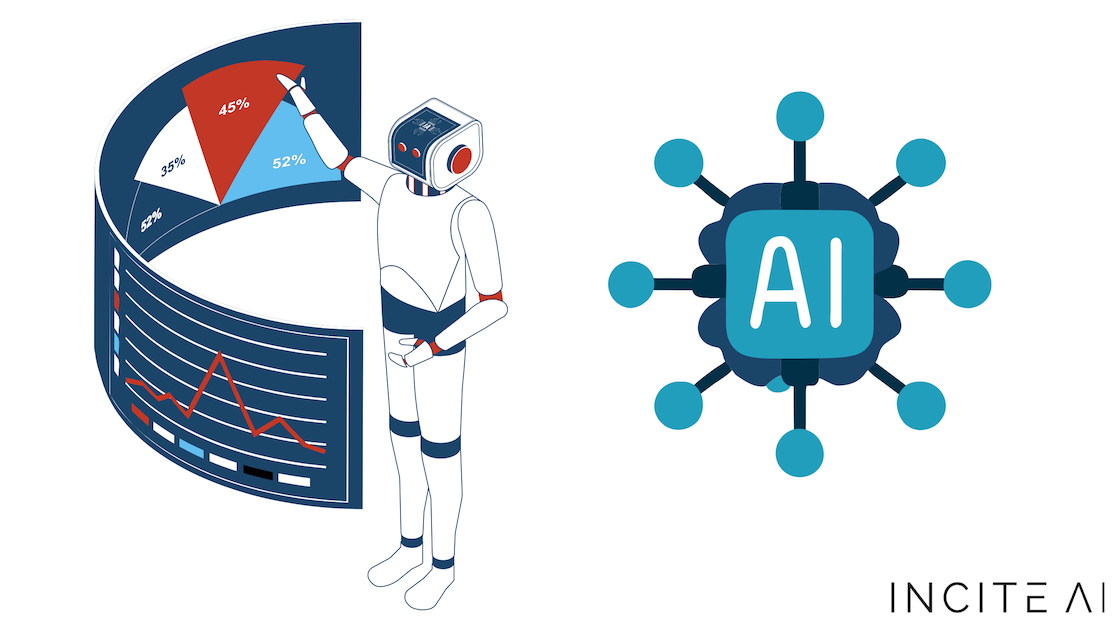